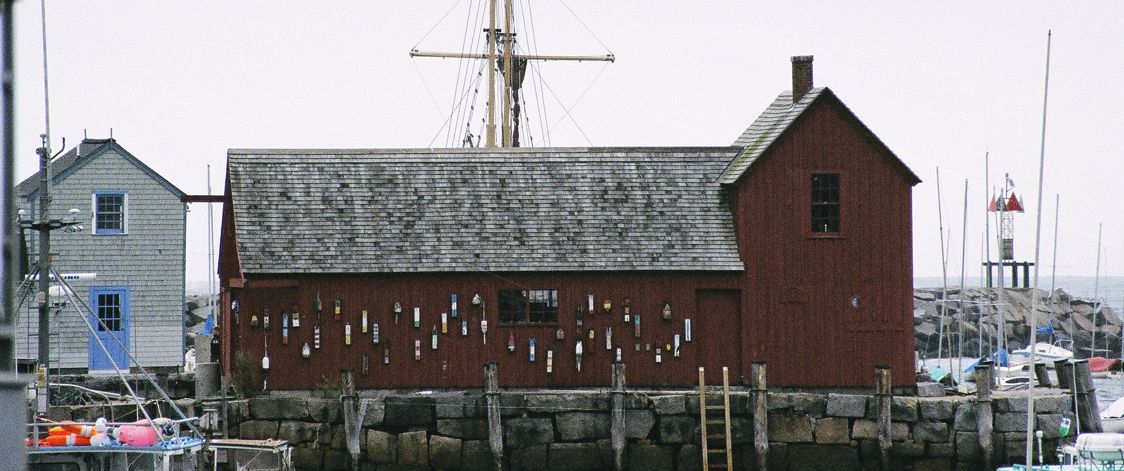
Motif No. 1, copyright © 2010 Douglas Cuthbertson
Asymptotic Notation
Big-O, Big-\(\Omega\) (Big-Omega), and Big-\(\Theta\) (Big-Theta) notation are used to describe some aspect of an algorithm (usually the running time or the memory resources it needs) relative to the size of the input data.
- Big-O Notation
- describes the asymptotic upper bound. In other words, if \(f(n)\) describes the running time of an algorithm; \(f(n) \text{ is said to be } O(g(n))\) if there exists a positive constant \(C \text{ and } n_0\) such that \(0 \le f(n) \le c g(n) \text{ for all } n \ge n_0\).
- Big-\(\Omega\) Notation
- describes the asymptotic lower bound. In other words, if \(f(n)\) describes the running time of an algorithm; \(f(n) \text{ is said to be } \Omega(g(n))\) if there exists a positive constant \(C \text{ and } n_0\) such that \(0 \le cg(n) \le f(n) \text{ for all } n \ge n_0\).
- Big-\(\Theta\) Notation
- describes the running time or memory usage. It seems to exist only when \(O(g(n)) == \Omega(g(n))\). In other words, if \(f(n)\) is the running time of an algorithm, then \(f(n)\) is said to be \(\Theta(g(n))\) if \(f(n) == O(g(n)) \text{ and } f(n) == \Omega(g(n))\). Mathematically, \(0 \le f(n) \le C_{1}g(n) \text{ for } n \ge n_0 \text{ and } 0 \le C_{2}g(n) \le f(n) \text{ for } n \ge n_0\). These equations can be merged into \(0 \le C_{2}g(n) \le f(n) \le C_{1}g(n) \text{ for } n \ge n_0\). In other words, \(f(n)\) is sandwiched between \(C_{2}g(n) \text{ and } C_{1}g(n)\).
Here is a summary of these three asymptotic notations. They are used to express the computational complexity of an algorithm.
Big-O | Big-\(\Omega\) | Big-\(\Theta\) |
---|---|---|
The maximum rate of growth of an algorithm can be characterized as less than or equal to a given function. | The minimum rate of growth of an algorithm can be characterized as greater than or equal to a given function. | The rate of growth of an algorithm is equal to a given function. |
The algorithm’s asymptotic upper bound. | The algorithm’s asymptotic lower bound. | The algorithm has an asymptotically tight bound. |
Worst case or ceiling of the growth rate. | Best case or floor of the growth rate. | The worst case and best case of the growth rate is characterized by the same function or that they converge on said function. |